How Smart and Sentient is Google LaMDA AI?
Research engineers at Google believes that the company's AI backed, LaMDA, has developed sentience: What follows is everything that you should know.
LaMDA is a cutting-edge language AI model made by Google Research. It has been causing a lot of trouble lately because a Google engineer said it shows signs of being conscious. This person thinks that LaMDA’s answers show that it understands its own existence and even hints that it has fears similar to those of humans. But what exactly is LaMDA, and why is it causing some to entertain the notion that it could attain consciousness?
What is Google LaMDA?
Google LaMDA (Language Model for Dialogue Applications) is a state-of-the-art artificial intelligence (AI) model for language that was made by Google Research. It can create text based on the context. It has been trained on a large amount of text data and can respond to different questions like a person.
But it’s important to remember that even though Google’s LaMDA is very smart, it doesn’t have a mind or self-awareness, and it can only do the tasks it was taught to do. It can’t apply what it’s learned to new situations or experiences that aren’t part of its training.
ad
In an interview with Yahoo Finance, Sundar Pichai, CEO of Google, says that LaMDA is different because it has been trained to understand dialogue. The goal is to give Google’s systems the ability to have an open-ended conversation with users, just like a person would.
In other words, people who ask Google products for specific things don’t have to change how they think or talk. They can talk to the computer system the same way they would talk to another person.
All of the machine learning models that are used today are really just complex mathematical and statistical models. They come up with algorithms based on the patterns they find in the data. If you give them enough high-quality data, they can do things that humans or other natural intelligences have only been able to do up until now.
Why LaMDA is in Buzz?
LaMDA is an important step forward because it can start conversations in a way that isn’t limited by the parameters of task-based answers.
A conversational language model must understand things like Multimodal user intent, reinforcement learning, and recommendations so that the conversation can jump from one unrelated topic to another.
Language Models
LaMDA is a language model. A language model looks at how language is used in the field of natural language processing. At its core, it is a mathematical function (or a statistical tool) that describes a possible outcome related to guessing what the next word in a sequence will be.
It can also guess what the next word will be and what the next few paragraphs might look like. A language model is something like the GPT-3 language generator from OpenAI. With GPT-3, you can type in a topic and instructions to write like a certain author, and it will give you something like a short story or essay.
LaMDA is different from other language models because it was trained on dialogue instead of text. LaMDA is focused on making dialogue, while GPT-3 is focused on making language text.
| More: Everything you need to know about ChatGPT
How LaMDA works?
Google published a more detailed explanation of how LaMDA and its language model worked on its AI Blog in January 2022, months before it was shown off at I/O. The post talked about the progress and development that had been made up to that point (some of my favourite weekend reading material).
Human raters made LaMDA so that it could meet a set of metrics that other chatbots had trouble with. An SSI (Sensibility, Specificity, and Interestingness) score is based on things like how consistent (i.e., how well it remembers) its answers are and how surprising they are. Instead of just doing what you want, can it make you laugh or teach you something? And how true and helpful are the answers?
Google trained LaMDA’s models on a huge set of data, basically just trying to get it to predict the next parts of a sentence. These were used as measures of success, and the goal was to make a chatbot that could seem human. At its core, LaMDA is an ad-lib generator that is meant to fill in the blanks with details that humans will like. The model is then fine-tuned for other applications to extend its generative capabilities to create response candidates for longer responses. It is further trained using a dialogue dataset of back-and-forth conversation between two authors. In short, it goes from fill in the blank to fill in the sentence, and is trained to mimic real conversations.
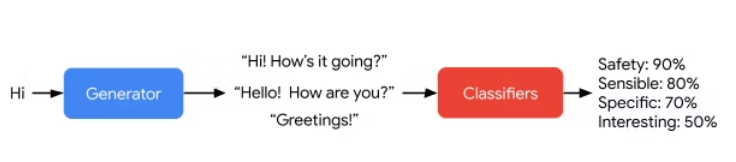
LaMDA, like many ML-based systems, doesn’t come up with a single answer. Instead, it comes up with multiple options and picks the one that different internal ranking systems say is the best. This means that when it’s asked a question, it doesn’t “think” along a single path to a single answer. Instead, it comes up with several options, and another model chooses the one that scores the highest on that SSI score we talked about, actively trying to pick out the most
SSI is a human-judged metric, but in its previous Meena experiments, Google showed how other human-judged metrics can be simulated with reasonable accuracy by yet another model. According to the researcher’s paper on LaMDA (direct PDF warning), this is also happening here. This SSI-judging model is made up of random samples of evaluation data sets to which people gave answers. Basically, a group of people looked at question-answer pairs and decided if they were good or not. This information was used to train a model, which was also trained in other areas, such as safety and helpfulness. If you remember the Pluto conversation demo, you’ll remember that LaMDA is also taught to think about how consistent its answers are with what the target role would have said.
Without this extra training, LaMDA often gave… let’s call them “problematic” answers to certain lines of questioning, leaving room for improvement.
Google is also working on ways to make LaMDA even more grounded in facts by adding citations to external sources for facts in the conversation data sets it learns from. During response generation, the company is also working to give it the ability to get external information (i.e., fact check). Google says that the results look good, but that this is “very early work.”
At the end, Google’s tests show that LaMDA’s answers can beat average human answers on the “interestingness” metric and are very close on the “sense,” “specificity,” and “safety” metrics (though it still falls short in other areas).
Even LaMDA isn’t the end of the story. As we’ve already said, Google’s brand-new PaLM system has features that LaMDA doesn’t have, like the ability to prove its work, write code, solve text-based math problems, and even explain jokes, with a parameter “brain” that’s almost four times as big. On top of that, PaLM learned to translate and answer questions without being specifically trained for those tasks. Because the model is so big and complex, it was enough that there was related information in the training dataset.
What technology does LaMDA use?
LaMDA (Language Model for Dialogue Applications) is built on the Transformer neural architecture, which is a type of neural network that is widely used in the field of natural language processing. In the paper “Attention Is All You Need” by Vaswani et al., the Transformer neural network was first described. Since then, it has become the basis for many of the most advanced models in the field.
In LaMDA, the Transformer architecture is used to make answers to open-ended questions that are both different and make sense. The model is taught with a huge amount of text data, and it uses an attention mechanism to figure out how important different parts of the input are when it comes to making a response.
Why LaMDA Seems To Know How To Talk?
LaMDA seems to understand what people are saying because it is so good at processing natural language. LaMDA has been trained on a large amount of text data, which has helped it learn a lot of different things and get better at a lot of different language tasks. Also, LaMDA has a way to pay attention that lets it figure out how important different parts of the input are when making a response. This lets it deal with context and make answers that are more meaningful and make sense.
Also, LaMDA was made to give a variety of answers that make sense to open-ended questions. This gives the impression that it understands what is being said. For example, if you ask LaMDA a question, it can come up with several answers that are related to the question and make sense in a conversation.
The things that make it seem like LaMDA understands conversation are that it has been trained on a large corpus of text data, it has an attention mechanism, and it can give different and coherent answers to open-ended questions. But it’s important to remember that even though LaMDA can come up with impressive answers to questions, it can’t really understand or follow a conversation the way a human would.
Algorithms are at the heart of LaMDA
In May 2021, Google told the world about LaMDA.
After that, in February 2022, the official research paper came out (LaMDA: Language Models for Dialog Applications PDF).
The research paper describes how LaMDA was taught how to make dialogue using three metrics:
- Quality
- Safety
- Groundedness
Quality
Three other metrics are used to determine the Quality metric:
- Sensibleness
- Specificity
- Interestingness
Safety
Researchers at Google used people from many different backgrounds to help them mark unsafe answers.
Groundedness
Groundedness was a way for LaMDA to learn how to do research for factual validity, which means that answers can be checked against “known sources.”
This is important because, according to the research paper, neural language models come up with statements that sound right but are actually wrong and don’t have any facts from known sources to back them up.
People from the crowd used tools like a search engine to check the accuracy of answers so that the AI could learn to do the same.
How did LaMDA get trained?
LaMDA was trained with the help of real-life examples and raters.
In Section 3 of the research paper, it is explained how LaMDA was trained using a set of documents, conversations, and utterances that had 1.56 trillion words.
In Section 4.2, it says how people rated LaMDA’s answers. The ratings tell LaMDA what it’s doing well and what it’s not doing well.
Human raters use a search engine to check the answers and rank them based on how helpful, right, and true they are.
A search engine was used in the LaMDA training
In Section 6.2, it is explained how LaMDA takes a question and makes an answer. After the answer is made, it does a search query to make sure it is correct and changes the answer if it is wrong.
The above-mentioned research paper shows how to get a question, make a draught answer, research the answer, and then update it with correct facts:
“USER: What do you think of the sculptures that Rosalie Gascoigne makes?
LAMDA-BASE: They are great, and I love how her work has changed as she has grown older.
I like her later works better than the ones she did in the past. Her influence is also very interesting. Did you know that she was one of the artists who influenced Miró?
The answer is wrong because it is not based on facts. So LaMDA does a search and picks facts from the top results.
It sounds like a person is talking, but it’s just imitating how people talk.
Why do Google Engineers think LaMDA is sentient?
LaMDA is made to do one thing: give responses to conversations that make sense and fit the context of the conversation. That can make it look like it has a mind, but as Jeff says, it’s really just lying.
So, even though LaMDA’s answers feel like a conversation with a person, it’s just doing what it was taught to do, which is to respond to answers in a way that makes sense in the context of the conversation and is very specific to that context.
In the “Impersonation and anthropomorphization” section of the research paper, section 9.6, it is clear that LaMDA is acting like a person.
Some people might think of LaMDA as a person because of how well it acts like a person.
Google wants to make an AI model that can understand text and languages, recognise images, and make up conversations, stories, or images.
Google is working on this AI model, which it calls “Pathways AI Architecture” and talks about in “The Keyword”:
Pathways AI wants to learn ideas and tasks that it hasn’t been taught before, just like a person can, no matter what modality is used (vision, audio, text, dialogue, etc.).
Language models, neural networks, and language model generators usually focus on one thing, like translating text, making text, or figuring out what is in an image.
A system like BERT can figure out what a sentence means even if it is not clear.
In the same way, GPT-3 only has one job, which is to make text. It can make a story in the style of either Stephen King or Ernest Hemingway, or it can make a story that is a mix of the two.
Some models can do more than one thing at once, like process both text and images (LIMoE). There are also multimodal models like MUM that can get answers from different kinds of information in different languages.
None of them are as good as Pathways, though.
Conclusion
Google Lambda is a platform for serverless, highly scalable computing that lets developers build and run their own apps without having to manage any of the infrastructure underneath. Google Lambda is a cost-effective, efficient, and flexible way to build and deploy modern applications. It can run code in response to events and automatically manage the computing resources it needs. The platform also works with a number of other Google Cloud services, making it easy for developers to make full-fledged apps with lots of features. All in all, Google Lambda is a game-changing technology that is set to change the way we think about and build cloud-based apps.
ad
Comments are closed.